Getting to know each other
Dec 18
/
Simon Shaw
In this month’s blog Mojave founder Simon Shaw chronicles the continuing story of his relationship with Open AI’s ChatGTP – are they getting to know one another better by now? Read on to find out.
My latest experiments with ChatGPT continue two lines of enquiry:
I’ll report on the first line of enquiry is in this blog. My next blog will report on the second.
- What does it do well and not so well?
- What won’t it tell us and why not?
I’ll report on the first line of enquiry is in this blog. My next blog will report on the second.
So: What does ChatGPT do well? Not so well?
Test question: How well does it synoptize?
‘Synoptize’ – an ugly as well as an uncommon word : only 0.03 occurrences per million words in modern English, thankfully. It’s the verb form of synopsis = “A brief or condensed statement presenting a combined or general view of something; a table, or set of paragraphs or headings, so arranged as to exhibit all the parts or divisions of a subject or work at one view” (Oxford English Dictionary).
I chose this as a test question partly because GPT suggested it:
Me: Which functions of ChatGPT should I select to answer the questions 'What does it do well?' and ‘What does it not do well?’
GPT: Does well in providing information on topics, generating concise summaries, and explaining concepts
Me: Is providing a synopsis the main (or one of the main) functions of AI using LLMs?
GPT: Indeed! When asked to provide a synopsis, an LLM can generate a concise summary that captures the essential information and assists users in quickly understanding the main points.
OK, while not exactly corresponding to the OED definition, I think this comes close enough to validate a test.
Method: I asked GPT around 100 questions using the same stem: ‘Which researchers and theorists are most closely associated with …’ [various well-known leadership “subjects”].
Results: GPT responded by giving me short answers summarising the information, always in the same format: an introductory paragraph, between 8 and 12 headings + short paragraphs, and a last paragraph more or less repeating the introduction. Most of the theorists and researchers it cited were known to me and would be familiar to anyone with a reasonable general knowledge of leadership and management. A high proportion of the same names were repeated in several different subjects.
For example, this is what happened when I picked motivation for the subject:
Me: Which researchers and theorists are most closely associated with motivation?
GPT: Maslow, Herzberg, Skinner, Pavlov … [+ 6 more, all known to me]
OK as a starting point. Really fast. Not wrong, as far as I can tell. But narrow and shallow.
Can it go wider?
Me: Which other theorists and researchers apart from the ones already mentioned can help us to understand motivation?
GPT: Ten more names: five familiar and relevant to the subject, three familiar but not very relevant, two new to me [thanks GPT!].
Can it go deeper?
Me: Which schools of thought do these theorists belong to?
GPT: Humanistic, behaviourist … [+ 6 more]
Me: What are the key differences between a humanistic theory of motivation and a behaviourist theory?
GPT: Focus on internal states vs external behaviour, free will vs determinism, innate goodness vs neutral … [+ 2 more and a summary]
Me: Can you sum up the differences between humanistic and behaviourist theories of motivation in a table?
GPT: Certainly! [Table a succinct summary of the differences + a new line saying how each theory critiques the other – didn’t ask for it but a good extra, thanks again!]
Yes to wide and deep, then – but how much deeper?
Me: How accurate is it to conclude that that humanistic theories see motivation as coming from inside whereas behaviourists see it as coming from outside?
GPT: Generally accurate as a broad characterisation but these perspectives have evolved over time, individual theorists within each camp have nuanced views, and contemporary theories often incorporate elements from both perspectives [my precis]
And how much wider?
Me: What light do recent discoveries in cognitive processes within the brain shed on motivation?
GPT: Recent discoveries in cognitive neuroscience have provided valuable insights into the neural processes underlying motivation [+ a short description of 8 discoveries – interesting!]
Me: What light do recent discoveries in cognitive processes within the brain shed on theories of motivation? [note the slight difference in this question from my previous one]
GPT: Recent discoveries provide neurobiological explanations and support for certain motivational processes and align with and enhance various motivational theories [+ 8 paragraphs connecting a theory to a discovery or vice versa + a suggestion: Integrating these neurobiological insights with psychological and behavioural perspectives can offer a more comprehensive understanding of the complex nature of motivation]
How about I ask it a hard question to see if it follows through on its own ‘thinking’?
Me: Does combining insights from these three perspectives – neurobiological, psychological and behavioural – produce a unified theory of motivation?
GPT: Achieving a truly unified theory of motivation is challenging [+ 7 reasons why it’s challenging]
Hmm, I think it ducked this one. Let’s see if it knows why:
Me: Would you classify this question as a 'hard' one, and what are the most relevant concepts of 'hard' in this context?
GPT: The classification of a question as "hard" in this context is subjective and can depend on various factors [+ 5 concepts of hard – complexity, ambiguity, interdisciplinary etc.]
That feels to me like another duck – a partial answer, almost as if it feels nervous! To be fair, I’d feel nervous if someone asked me a question about a unified theory of anything.
Conclusion: How well does it synoptize?
Reminder: “A brief or condensed statement presenting a combined or general view of something; a table, or set of paragraphs or headings, so arranged as to exhibit all the parts or divisions of a subject or work at one view”
Well
- Brief
- Condensed
- Combined
- General
- Paragraphs and headings
- Table
- At one view
Not so well
- All the parts etc.
Disclaimer:
it’s not a real test, I don’t pretend to be scientific or anything. But I’ve
learned three things from doing it:
More on that in my next blog …
- First queries to GTP are just that – first not last. GTP’s response is a starter for 10 (yes, it usually is 10!)
- Make your follow-up queries wider and deeper – use the ‘four flavours’ of follow-up: 1) Elaboration (Tell me more about …), 2) Different perspective (What about …?), 3) Connective (How does …?), 4) Challenge (Why …?).
- Sooner or later, you’ll come to the end of what GPT can – or is prepared – to tell you. However you change the query you come up against the wall.
More on that in my next blog …
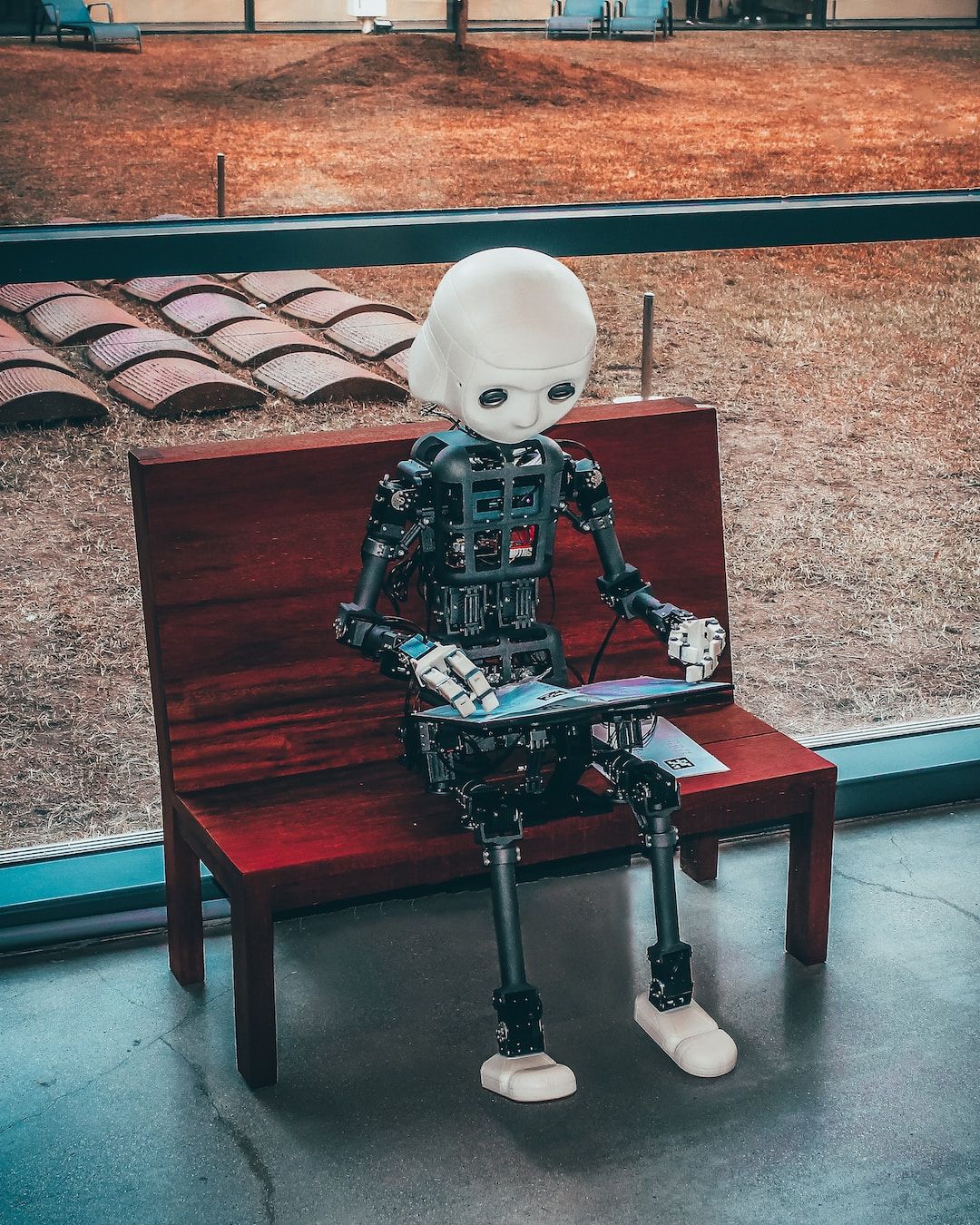
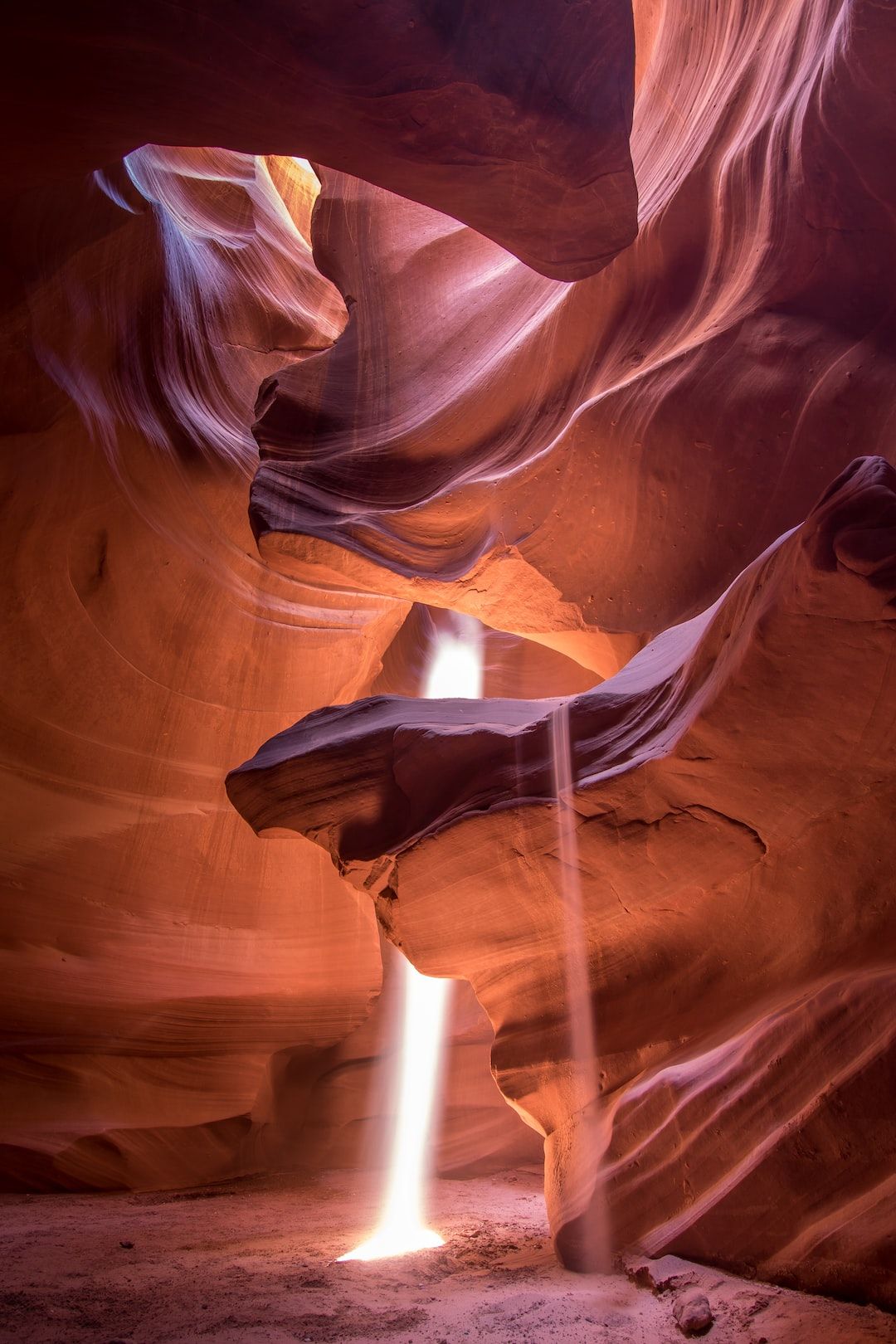
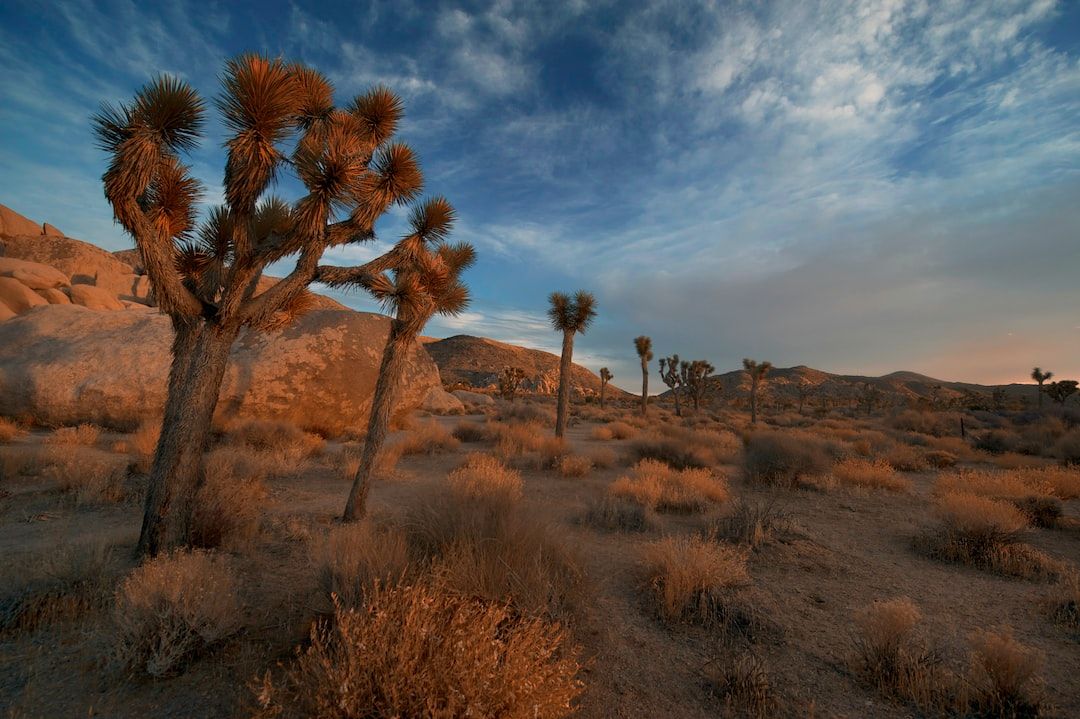
Please contact me!
Please get in touch with more information about the Mojave Strategy Expedition Programme, and show me how my organisation can emerge stronger.
Thank you!
Karen Goldring FCIPD
Karen had an extensive and hugely successful career in strategic HR before becoming a leadership coach. From rapid growth SMEs to large global corporates, Karen has gained immensely valuable insights and a thorough understanding of what it takes to make people and their teams high performing. She has particular experience working within fast paced tech companies.
A qualified Insights Practitioner - one of the leadership models we wholly rate here at Mojave due to its pragmatism and relevance to lived experience - Karen offers teams and their members the ability to really understand themselves and each other. From the informed start point that results from the Insights process, Karen will work with you to identify the objectives that matter most to you and your organisation, and ensure you get there.
Click below to learn more about Karen and whether she’s the right kind of coach for you..
Piers Mummery
He has
built, grown, sold, bought funded and capitalised a whole range of businesses,
and he’s learned loads in the process. One of the things he’s learned is
that he loves helping others do the same.
As he says on his website, there are no magic answers, but applying a few basic fundamentals well, consistently and in the right way for you and your business is key. And it’s that acknowledgement that the right way will vary for each of us that means we are proud to work with Piers as a coach who is totally aligned with our own values and philosophy.
As he says on his website, there are no magic answers, but applying a few basic fundamentals well, consistently and in the right way for you and your business is key. And it’s that acknowledgement that the right way will vary for each of us that means we are proud to work with Piers as a coach who is totally aligned with our own values and philosophy.
Click below to learn more about Piers and whether he’s the right kind of coach for you..
Rachel Smith
Formerly an architect, Rachel herself began to realise that she could process and articulate her own ideas far more effectively through visuals. What began as a process to enable her to be more effective in her own career soon evolved into a process that could help others exceed in theirs.
As an accredited executive coach and visual thinking teacher, Rachel’s incredible skills enable her to draw the thoughts and ideas her clients articulate. Being able to ‘see what you say’ as you work through each session can be incredibly powerful in enabling you to identify new connections or blockers that can otherwise remain hidden.
Click below to learn more about Rachel and whether she’s the right kind of coach for you..
Write your awesome label here.
Notify me!
When the next series of Leading People online workshop dates are released.
Thank you!
Leading Operations
Thank you!
Write your awesome label here.